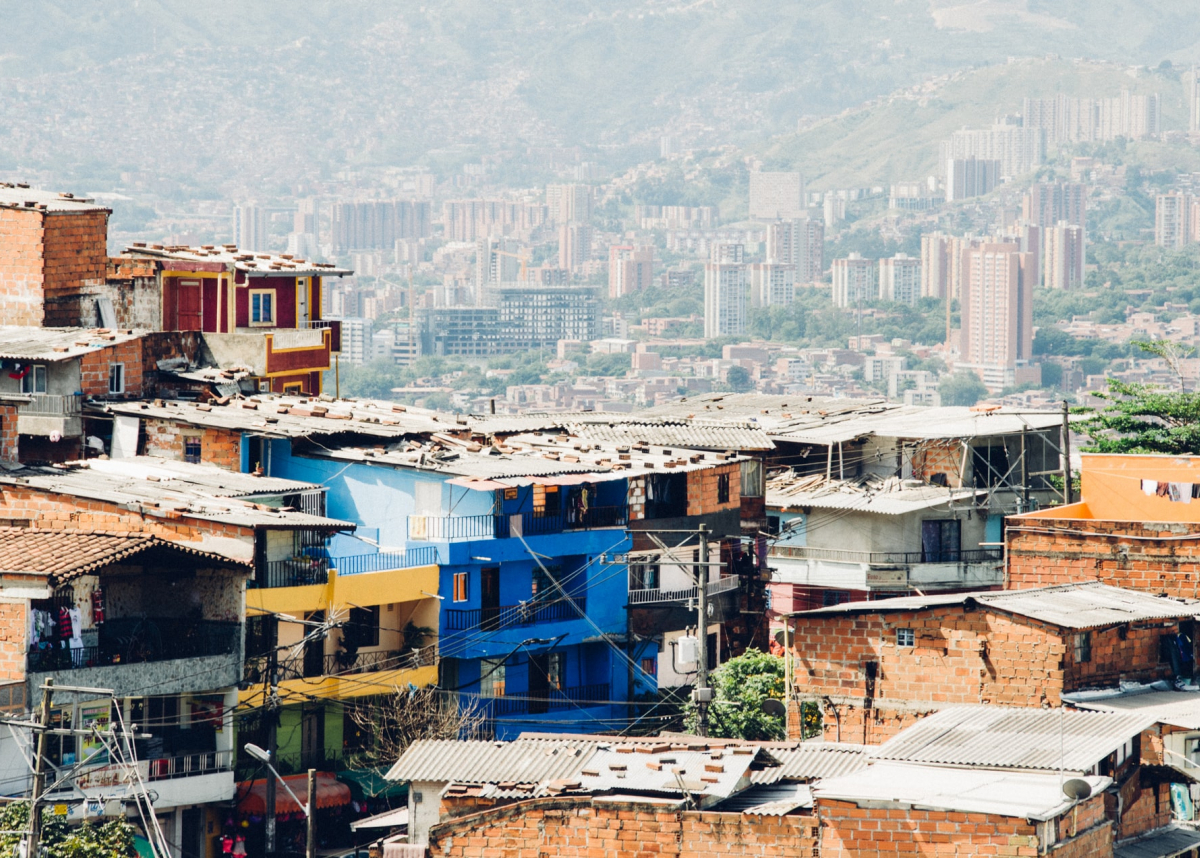
Colombia has the second-highest level of inequality in the Latin America region. Mauricio Quiñones Domínguez and Lina Martínez look at how social protection measures like subsidies are now out of date.
As part of PEAK Urban, I am currently leading a research project that aims to model the urban footprint growth of selected Latin American Cities (LAC). The main premise in this project is that if we can predict what the urban footprint is going to look like in the future, we can better advise policymakers and stakeholders. So that they can plan the required infrastructure and core services, and take the necessary actions for maximizing the benefits of agglomeration and at the same time minimizing the adverse impacts of urban growth itself.
At EAFIT, we decided to tackle the urban footprint growth project using two principles. Firstly, we use open data to enable other researchers to apply our model and methodologies to their contexts. Secondly, we use a flexible and simple model with good explanatory power (i.e: a parsimonious model) that can be adapted to the reality of different cities. These model attributes are important as not all cities have the same available data, set of growth drivers, environmental risks, protected areas, or growth dynamics. Overall, we conceive the urban footprint growth model as an important component of a larger and more complex feedback-planning system, which will help us acquire new insights for taking more sustainable actions.
We chose a data-driven approach based on machine learning algorithms to build our urban footprint growth model. Machine learning is a useful tool for dealing with data, and there are many successful examples of its application in domains as diverse as medicine, economics, entertainment, and engineering. It has been used for understanding data distributions (e.g. income within a population group), extracting clusters (e.g. regionalization), creating models for predicting continuous outputs (e.g. estimating pollution or GDP), and assigning finite class labels to inputs (e.g. geo-demographic classifiers and urban/rural classifiers from satellite imagery), among many others.
However, many machine learning algorithms do not provide an understanding beyond correlation and therefore they are not well suited for solving intervention or counterfactual inquiries since there is no insight into causality. So, it is worth asking ourselves, what techniques are professionals in the social sciences using for including causality in their data analyses? How can we incorporate causality in machine learning? How could the enhancements derived from including causality in our models positively influence our capabilities within PEAK Urban?
Economists have used analytical techniques to address the issue of causality, always from theoretical models. Some of these analytical techniques include Granger’s causality in time series, the difference in differences, analysis of natural experiments, instrumental variables techniques, regression in discontinuities, and randomized experiments. All these techniques have advantages and challenges such as the lack of external validity for natural experiments, setting up randomized experiments at different scales, and including budget and ethical constraints, among others.
Professor Judea Pearl, a Turing Award recipient, shows that it is possible to include causality in data-driven research through what he calls a structural causal model (SCM). An SCM has three parts, a graphical model made of nodes connected by directed edges, a counterfactual and interventional logic, and a set of structural equations. The first part of the SCM encodes the expert knowledge, while the second part represents the queries that the researcher wants to answer, and the third part provides the semantics for joining the two previous parts with a mathematical language. The required engine for solving the SCM given the data has been refined in the last three decades or so, but it still needs to reach a larger audience, particularly in urban science.
Pearl classifies the inference in causal reasoning within a three-level hierarchy with increasing complexity and analytic power, corresponding to 1. associations, 2. interventions, and 3. counterfactuals. He argues that the capability of a machine learning system to perform causal inference increases from zero in the association level - independent of the amount of data in the experiment – to the ability to reason about the future outcomes of current actions in the association level, to the most complex causal inference analysis capability in the counterfactual level, where the algorithm is able to “reflect” and “imagine” the outcomes that could have obtained had it taken different actions in the past. A machine learning system in a higher level in the hierarchy can perform tasks associated to lower levels, but the opposite is not feasible, which means that machine learning systems framed in lower levels can’t perform inference tasks associated to higher levels.
The association capability in level one of Pearl’s hierarchy encloses most of the current machine learning applications in prediction, diagnosis, and recognition because they do not consider interventions or counterfactuals. Applications that rely on associations have had a significant impact on urban science and spatial economics during the last couple of decades, and we can’t underestimate them.
However, they don’t provide solid foundations for answering policymaker’s questions such as, what will happen in the future if I take action X now? Or counterfactual questions that the public might have such as “what would the present look like had the policymaker taken action X in the past?” For instance, what would have happened to air pollution in major Colombian cities, if in the 1990s the government had adopted and enforced the European standards for vehicle emissions? We believe that counterfactuals can be a useful approach in the urban planning realm, preventing us from making the same mistakes again. They can help urban planners and local governments reconsider their proposals and think about long-term impacts, and the results of their actions in the future.
We have been collecting and cleaning the input data for our project, and designing a suitable spatio-temporal machine-learning model that can ingest and process multiple variables. As the project evolves, we aim to frame our urban footprint growth model within the causal intervention level from Pearl’s hierarchy. This will enable policymakers and stakeholders to assess “what if” scenarios and answer intervention questions. Some examples include;
By thinking about intervention and counterfactual queries and solving them with “causality-aware” data-driven algorithms, cities will be better equipped to plan and assess urban policies. Including causality in urban contexts is not always feasible, and can be challenging. However, the causality theory combined with new tools and interdisciplinary work will help us to get better insights from data, and to improve our prediction models for planning the transition towards a more sustainable urban future.